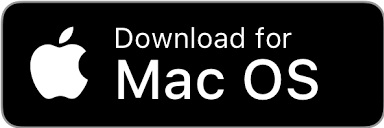
I am interested in the statistical power of the predictors' effects on the dependent variable. My dataset has 98 respondents and I have 7 constructs predicting one specific dependent variable. I have a problem relating to the statistical power and critical t boundaries for f square values (effect size). Great to see that there is a thread on G*power here already. With five predictors, this would require you to have a sample size of 248 for a power of 95% to find the 0.2 coefficient significant. If you have an expectation for the standardized coefficient of 0.2 and an overall R² of 0.25, you would get an effect size f² of 0.053. It would require a standardized coefficient of roughly 0.275 if your overall R² is at about 0.5 and a standardized coefficient of 0.336 if your overall R² is at 0.25. Unfortunately, this effect size is not very straightforward to determine. It is the contribution to the R² by the predictor (R²inlcuded - R²excluded) / (1 - R²included). There you have to set the effect size f², which is the same effect size that is reported in SmartPLS under f². Hence, you might want to choose the "Linear multiple regression: Fixed model, single regression coefficient" as the method in G*Power. Many researchers are more interested in the significance of single effects instead of the variance explained by the overall regression equation. Hence, with 138 cases you will find a model with an R² of 0.15 significant at 95% of the time.
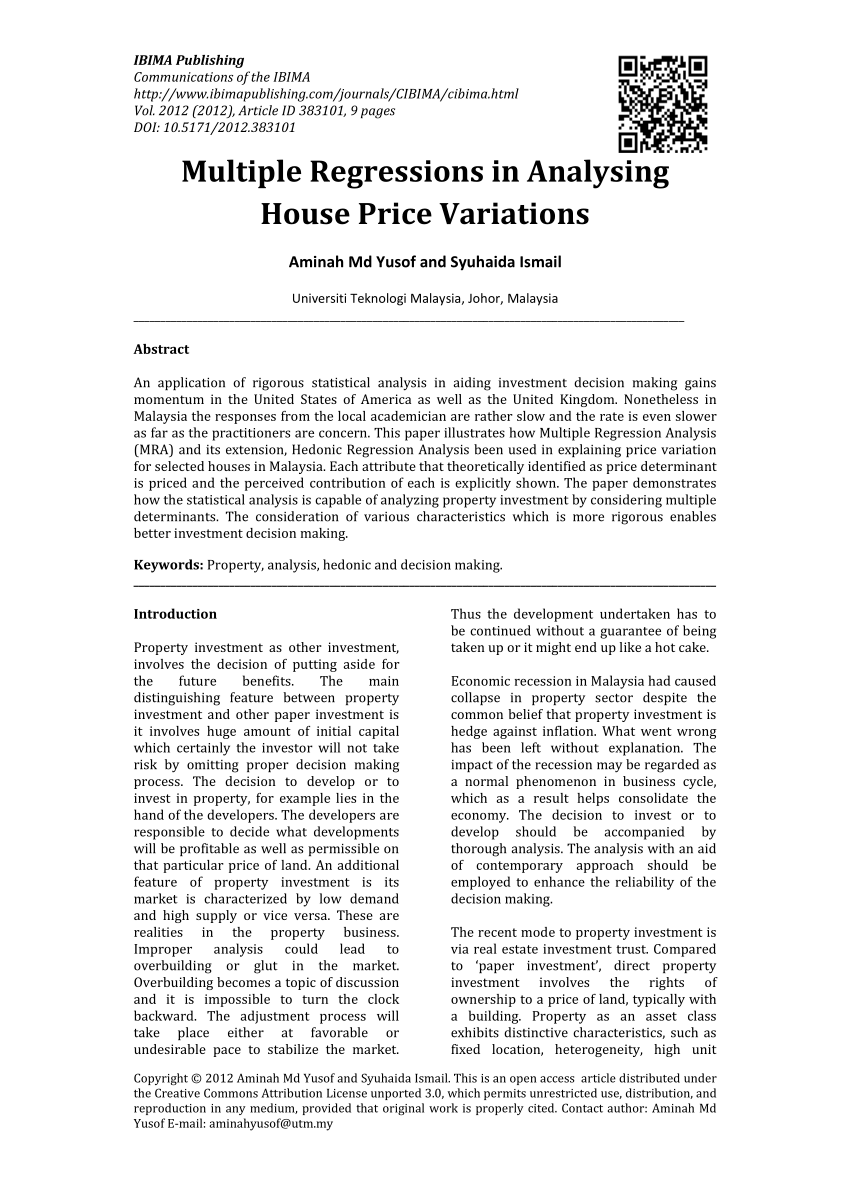

It assesses the significance of the R² at the given effect size level (e.g., effects size 0.15 = R²). The test above is for the F test of a regression. The questions is, which test is the one you are interested in.
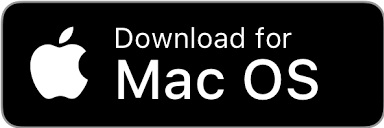